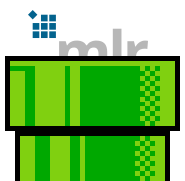
Wrap a Learner into a PipeOp to to predict multiple Quantiles
Source:R/PipeOpLearnerQuantiles.R
mlr_pipeops_learner_quantiles.Rd
Wraps a LearnerRegr
into a PipeOp
to predict multiple quantiles.
PipeOpLearnerQuantiles
only supports LearnerRegr
s that have quantiles
as a possible pedict_type
.
It produces quantile-based predictions for multiple quantiles in one PredictionRegr
. This is especially helpful if the LearnerRegr
can only predict one quantile (like for example LearnerRegrGBM
in mlr3extralearners
)
Inherits the $param_set
(and therefore $param_set$values
) from the Learner
it is constructed from.
Construction
learner
::Learner
|character(1)
Learner
to wrap, or a string identifying aLearner
in themlr3::mlr_learners
Dictionary
. TheLearner
has to be aLearnerRegr
withpredict_type
"quantiles"
. This argument is always cloned; to access theLearner
insidePipeOpLearnerQuantiles
by-reference, use$learner
.id
::character(1)
Identifier of the resulting object, internally defaulting to theid
of theLearner
being wrapped.param_vals
:: namedlist
List of hyperparameter settings, overwriting the hyperparameter settings that would otherwise be set during construction. Defaultlist()
.
Input and Output Channels
PipeOpLearnerQuantiles
has one input channel named "input"
, taking a TaskRegr
specific to the Learner
type given to learner
during construction; both during training and prediction.
PipeOpLearnerQuantiles
has one output channel named "output"
, producing NULL
during training and a PredictionRegr
object
during prediction.
The output during prediction is a PredictionRegr
on the prediction input data that aggregates all result
s produced by the Learner
for each quantile in quantiles
.
trained on the training input data.
State
The $state
is set during training. It is a named list
with the member:
model_states
::list
List of the states of all models created by theLearner
's$.train()
function.
Parameters
The parameters are exactly the parameters of the Learner
wrapped by this object.
q_vals
::numeric
Quantiles to use for training and prediction. Initialized toc(0.05, 0.5, 0.95)
q_response
::numeric(1)
Which quantile inquantiles
to use as aresponse
for thePredictionRegr
during prediction. Initialized to0.5
.
Fields
Fields inherited from PipeOp
, as well as:
learner
::LearnerRegr
Learner
that is being wrapped. Read-only.learner_model
::Learner
IfPipeOpLearnerQuantiles
has been trained, this is alist
containing theLearner
s for each quantile. Otherwise, this contains theLearner
that is being wrapped. Read-only.predict_type
::character(1)
Predict type of thePipeOpLearnerQuantiles
, which is always"response" "quantiles"
.
Methods
Methods inherited from PipeOp
.
See also
https://mlr-org.com/pipeops.html
Other PipeOps:
PipeOp
,
PipeOpEncodePL
,
PipeOpEnsemble
,
PipeOpImpute
,
PipeOpTargetTrafo
,
PipeOpTaskPreproc
,
PipeOpTaskPreprocSimple
,
mlr_pipeops
,
mlr_pipeops_adas
,
mlr_pipeops_blsmote
,
mlr_pipeops_boxcox
,
mlr_pipeops_branch
,
mlr_pipeops_chunk
,
mlr_pipeops_classbalancing
,
mlr_pipeops_classifavg
,
mlr_pipeops_classweights
,
mlr_pipeops_colapply
,
mlr_pipeops_collapsefactors
,
mlr_pipeops_colroles
,
mlr_pipeops_copy
,
mlr_pipeops_datefeatures
,
mlr_pipeops_decode
,
mlr_pipeops_encode
,
mlr_pipeops_encodeimpact
,
mlr_pipeops_encodelmer
,
mlr_pipeops_encodeplquantiles
,
mlr_pipeops_encodepltree
,
mlr_pipeops_featureunion
,
mlr_pipeops_filter
,
mlr_pipeops_fixfactors
,
mlr_pipeops_histbin
,
mlr_pipeops_ica
,
mlr_pipeops_imputeconstant
,
mlr_pipeops_imputehist
,
mlr_pipeops_imputelearner
,
mlr_pipeops_imputemean
,
mlr_pipeops_imputemedian
,
mlr_pipeops_imputemode
,
mlr_pipeops_imputeoor
,
mlr_pipeops_imputesample
,
mlr_pipeops_kernelpca
,
mlr_pipeops_learner
,
mlr_pipeops_learner_pi_cvplus
,
mlr_pipeops_missind
,
mlr_pipeops_modelmatrix
,
mlr_pipeops_multiplicityexply
,
mlr_pipeops_multiplicityimply
,
mlr_pipeops_mutate
,
mlr_pipeops_nearmiss
,
mlr_pipeops_nmf
,
mlr_pipeops_nop
,
mlr_pipeops_ovrsplit
,
mlr_pipeops_ovrunite
,
mlr_pipeops_pca
,
mlr_pipeops_proxy
,
mlr_pipeops_quantilebin
,
mlr_pipeops_randomprojection
,
mlr_pipeops_randomresponse
,
mlr_pipeops_regravg
,
mlr_pipeops_removeconstants
,
mlr_pipeops_renamecolumns
,
mlr_pipeops_replicate
,
mlr_pipeops_rowapply
,
mlr_pipeops_scale
,
mlr_pipeops_scalemaxabs
,
mlr_pipeops_scalerange
,
mlr_pipeops_select
,
mlr_pipeops_smote
,
mlr_pipeops_smotenc
,
mlr_pipeops_spatialsign
,
mlr_pipeops_subsample
,
mlr_pipeops_targetinvert
,
mlr_pipeops_targetmutate
,
mlr_pipeops_targettrafoscalerange
,
mlr_pipeops_textvectorizer
,
mlr_pipeops_threshold
,
mlr_pipeops_tomek
,
mlr_pipeops_tunethreshold
,
mlr_pipeops_unbranch
,
mlr_pipeops_updatetarget
,
mlr_pipeops_vtreat
,
mlr_pipeops_yeojohnson
Other Meta PipeOps:
mlr_pipeops_learner
,
mlr_pipeops_learner_cv
,
mlr_pipeops_learner_pi_cvplus
Examples
library("mlr3")
task = tsk("boston_housing")
learner = lrn("regr.debug")
po = mlr_pipeops$get("learner_quantiles", learner)
po$train(list(task))
#> $output
#> NULL
#>
po$predict(list(task))
#> $output
#> <PredictionRegr> for 506 observations:
#> row_ids truth q0.05 q0.5 q0.95 response
#> 1 24.0 10.2 21.2 43.4 21.2
#> 2 21.6 10.2 21.2 43.4 21.2
#> 3 34.7 10.2 21.2 43.4 21.2
#> --- --- --- --- --- ---
#> 504 23.9 10.2 21.2 43.4 21.2
#> 505 22.0 10.2 21.2 43.4 21.2
#> 506 19.0 10.2 21.2 43.4 21.2
#>