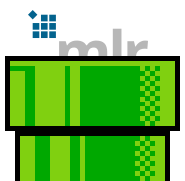
Tune the Threshold of a Classification Prediction
Source:R/PipeOpTuneThreshold.R
mlr_pipeops_tunethreshold.Rd
Tunes optimal probability thresholds over different PredictionClassif
s.
mlr3::Learner
predict_type
: "prob"
is required.
Thresholds for each learner are optimized using the Optimizer
supplied via
the param_set
.
Defaults to GenSA
.
Returns a single PredictionClassif
.
This PipeOp should be used in conjunction with PipeOpLearnerCV
in order to
optimize thresholds of cross-validated predictions.
In order to optimize thresholds without cross-validation, use PipeOpLearnerCV
in conjunction with ResamplingInsample
.
Format
R6Class
object inheriting from PipeOp
.
Construction
* `PipeOpTuneThreshold$new(id = "tunethreshold", param_vals = list())` \cr
(`character(1)`, `list`) -> `self` \cr
id
::character(1)
Identifier of resulting object. Default: "tunethreshold".param_vals
:: namedlist
List of hyperparameter settings, overwriting the hyperparameter settings that would otherwise be set during construction. Defaultlist()
.
Input and Output Channels
Input and output channels are inherited from PipeOp
.
Parameters
The parameters are the parameters inherited from PipeOp
, as well as:
measure
::Measure
|character
Measure
to optimize for. Will be converted to aMeasure
in case it ischaracter
. Initialized to"classif.ce"
, i.e. misclassification error.optimizer
::Optimizer
|character(1)
Optimizer
used to find optimal thresholds. Ifcharacter
, converts toOptimizer
viaopt
. Initialized toOptimizerGenSA
.log_level
::character(1)
|integer(1)
Set a temporary log-level forlgr::get_logger("bbotk")
. Initialized to: "warn".
Internals
Uses the optimizer
provided as a param_val
in order to find an optimal threshold.
See the optimizer
parameter for more info.
Methods
Only methods inherited from PipeOp
.
See also
https://mlr-org.com/pipeops.html
Other PipeOps:
PipeOp
,
PipeOpEnsemble
,
PipeOpImpute
,
PipeOpTargetTrafo
,
PipeOpTaskPreproc
,
PipeOpTaskPreprocSimple
,
mlr_pipeops
,
mlr_pipeops_boxcox
,
mlr_pipeops_branch
,
mlr_pipeops_chunk
,
mlr_pipeops_classbalancing
,
mlr_pipeops_classifavg
,
mlr_pipeops_classweights
,
mlr_pipeops_colapply
,
mlr_pipeops_collapsefactors
,
mlr_pipeops_colroles
,
mlr_pipeops_copy
,
mlr_pipeops_datefeatures
,
mlr_pipeops_encode
,
mlr_pipeops_encodeimpact
,
mlr_pipeops_encodelmer
,
mlr_pipeops_featureunion
,
mlr_pipeops_filter
,
mlr_pipeops_fixfactors
,
mlr_pipeops_histbin
,
mlr_pipeops_ica
,
mlr_pipeops_imputeconstant
,
mlr_pipeops_imputehist
,
mlr_pipeops_imputelearner
,
mlr_pipeops_imputemean
,
mlr_pipeops_imputemedian
,
mlr_pipeops_imputemode
,
mlr_pipeops_imputeoor
,
mlr_pipeops_imputesample
,
mlr_pipeops_kernelpca
,
mlr_pipeops_learner
,
mlr_pipeops_missind
,
mlr_pipeops_modelmatrix
,
mlr_pipeops_multiplicityexply
,
mlr_pipeops_multiplicityimply
,
mlr_pipeops_mutate
,
mlr_pipeops_nmf
,
mlr_pipeops_nop
,
mlr_pipeops_ovrsplit
,
mlr_pipeops_ovrunite
,
mlr_pipeops_pca
,
mlr_pipeops_proxy
,
mlr_pipeops_quantilebin
,
mlr_pipeops_randomprojection
,
mlr_pipeops_randomresponse
,
mlr_pipeops_regravg
,
mlr_pipeops_removeconstants
,
mlr_pipeops_renamecolumns
,
mlr_pipeops_replicate
,
mlr_pipeops_scale
,
mlr_pipeops_scalemaxabs
,
mlr_pipeops_scalerange
,
mlr_pipeops_select
,
mlr_pipeops_smote
,
mlr_pipeops_spatialsign
,
mlr_pipeops_subsample
,
mlr_pipeops_targetinvert
,
mlr_pipeops_targetmutate
,
mlr_pipeops_targettrafoscalerange
,
mlr_pipeops_textvectorizer
,
mlr_pipeops_threshold
,
mlr_pipeops_unbranch
,
mlr_pipeops_updatetarget
,
mlr_pipeops_vtreat
,
mlr_pipeops_yeojohnson
Examples
if (requireNamespace("bbotk")) {
if (requireNamespace("rpart")) {
library("mlr3")
task = tsk("iris")
pop = po("learner_cv", lrn("classif.rpart", predict_type = "prob")) %>>%
po("tunethreshold")
task$data()
pop$train(task)
pop$state
}
}
#> $classif.rpart
#> $classif.rpart$model
#> n= 150
#>
#> node), split, n, loss, yval, (yprob)
#> * denotes terminal node
#>
#> 1) root 150 100 setosa (0.33333333 0.33333333 0.33333333)
#> 2) Petal.Length< 2.45 50 0 setosa (1.00000000 0.00000000 0.00000000) *
#> 3) Petal.Length>=2.45 100 50 versicolor (0.00000000 0.50000000 0.50000000)
#> 6) Petal.Width< 1.75 54 5 versicolor (0.00000000 0.90740741 0.09259259) *
#> 7) Petal.Width>=1.75 46 1 virginica (0.00000000 0.02173913 0.97826087) *
#>
#> $classif.rpart$log
#> Empty data.table (0 rows and 3 cols): stage,class,msg
#>
#> $classif.rpart$train_time
#> [1] 0.003
#>
#> $classif.rpart$param_vals
#> $classif.rpart$param_vals$xval
#> [1] 0
#>
#>
#> $classif.rpart$task_hash
#> [1] "b39ef23a66b1f1ee"
#>
#> $classif.rpart$feature_names
#> [1] "Petal.Length" "Petal.Width" "Sepal.Length" "Sepal.Width"
#>
#> $classif.rpart$mlr3_version
#> [1] ‘0.18.0’
#>
#> $classif.rpart$data_prototype
#> Empty data.table (0 rows and 5 cols): Species,Petal.Length,Petal.Width,Sepal.Length,Sepal.Width
#>
#> $classif.rpart$task_prototype
#> Empty data.table (0 rows and 5 cols): Species,Petal.Length,Petal.Width,Sepal.Length,Sepal.Width
#>
#> $classif.rpart$train_task
#> <TaskClassif:iris> (150 x 5): Iris Flowers
#> * Target: Species
#> * Properties: multiclass
#> * Features (4):
#> - dbl (4): Petal.Length, Petal.Width, Sepal.Length, Sepal.Width
#>
#> $classif.rpart$affected_cols
#> [1] "Petal.Length" "Petal.Width" "Sepal.Length" "Sepal.Width"
#>
#> $classif.rpart$intasklayout
#> Key: <id>
#> id type
#> <char> <char>
#> 1: Petal.Length numeric
#> 2: Petal.Width numeric
#> 3: Sepal.Length numeric
#> 4: Sepal.Width numeric
#>
#> $classif.rpart$outtasklayout
#> Key: <id>
#> id type
#> <char> <char>
#> 1: classif.rpart.prob.setosa numeric
#> 2: classif.rpart.prob.versicolor numeric
#> 3: classif.rpart.prob.virginica numeric
#>
#> $classif.rpart$outtaskshell
#> Empty data.table (0 rows and 4 cols): Species,classif.rpart.prob.setosa,classif.rpart.prob.versicolor,classif.rpart.prob.virginica
#>
#>
#> $tunethreshold
#> $tunethreshold$threshold
#> setosa versicolor virginica
#> 0.5134816 0.8486673 0.2710435
#>
#>