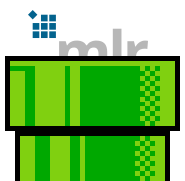
Linearly Transform a Numeric Target to Match Given Boundaries
Source:R/PipeOpTrafo.R
mlr_pipeops_targettrafoscalerange.Rd
Linearly transforms a numeric target of a TaskRegr
so it is between lower
and upper
. The formula for this is \(x' = offset + x * scale\),
where \(scale\) is \((upper - lower) / (max(x) - min(x))\) and
\(offset\) is \(-min(x) * scale + lower\). The same transformation is applied during training and
prediction.
Format
R6Class
object inheriting from PipeOpTargetTrafo
/PipeOp
Construction
id
::character(1)
Identifier of resulting object, default"targettrafoscalerange"
.param_vals
:: namedlist
List of hyperparameter settings, overwriting the hyperparameter settings that would otherwise be set during construction. Defaultlist()
.
Input and Output Channels
Input and output channels are inherited from PipeOpTargetTrafo
.
Parameters
The parameters are the parameters inherited from PipeOpTargetTrafo
, as well as:
lower
::numeric(1)
Target value of smallest item of input target. Initialized to 0.upper
::numeric(1)
Target value of greatest item of input target. Initialized to 1.
Internals
Overloads PipeOpTargetTrafo
's .get_state()
, .transform()
, and
.invert()
. Should be used in combination with PipeOpTargetInvert
.
Methods
Only methods inherited from PipeOpTargetTrafo
/PipeOp
.
See also
https://mlr-org.com/pipeops.html
Other PipeOps:
PipeOp
,
PipeOpEnsemble
,
PipeOpImpute
,
PipeOpTargetTrafo
,
PipeOpTaskPreproc
,
PipeOpTaskPreprocSimple
,
mlr_pipeops
,
mlr_pipeops_boxcox
,
mlr_pipeops_branch
,
mlr_pipeops_chunk
,
mlr_pipeops_classbalancing
,
mlr_pipeops_classifavg
,
mlr_pipeops_classweights
,
mlr_pipeops_colapply
,
mlr_pipeops_collapsefactors
,
mlr_pipeops_colroles
,
mlr_pipeops_copy
,
mlr_pipeops_datefeatures
,
mlr_pipeops_encode
,
mlr_pipeops_encodeimpact
,
mlr_pipeops_encodelmer
,
mlr_pipeops_featureunion
,
mlr_pipeops_filter
,
mlr_pipeops_fixfactors
,
mlr_pipeops_histbin
,
mlr_pipeops_ica
,
mlr_pipeops_imputeconstant
,
mlr_pipeops_imputehist
,
mlr_pipeops_imputelearner
,
mlr_pipeops_imputemean
,
mlr_pipeops_imputemedian
,
mlr_pipeops_imputemode
,
mlr_pipeops_imputeoor
,
mlr_pipeops_imputesample
,
mlr_pipeops_kernelpca
,
mlr_pipeops_learner
,
mlr_pipeops_missind
,
mlr_pipeops_modelmatrix
,
mlr_pipeops_multiplicityexply
,
mlr_pipeops_multiplicityimply
,
mlr_pipeops_mutate
,
mlr_pipeops_nmf
,
mlr_pipeops_nop
,
mlr_pipeops_ovrsplit
,
mlr_pipeops_ovrunite
,
mlr_pipeops_pca
,
mlr_pipeops_proxy
,
mlr_pipeops_quantilebin
,
mlr_pipeops_randomprojection
,
mlr_pipeops_randomresponse
,
mlr_pipeops_regravg
,
mlr_pipeops_removeconstants
,
mlr_pipeops_renamecolumns
,
mlr_pipeops_replicate
,
mlr_pipeops_scale
,
mlr_pipeops_scalemaxabs
,
mlr_pipeops_scalerange
,
mlr_pipeops_select
,
mlr_pipeops_smote
,
mlr_pipeops_spatialsign
,
mlr_pipeops_subsample
,
mlr_pipeops_targetinvert
,
mlr_pipeops_targetmutate
,
mlr_pipeops_textvectorizer
,
mlr_pipeops_threshold
,
mlr_pipeops_tunethreshold
,
mlr_pipeops_unbranch
,
mlr_pipeops_updatetarget
,
mlr_pipeops_vtreat
,
mlr_pipeops_yeojohnson
Examples
if (requireNamespace("rpart")) {
library(mlr3)
task = tsk("boston_housing")
po = PipeOpTargetTrafoScaleRange$new()
po$train(list(task))
po$predict(list(task))
#syntactic sugar for a graph using ppl():
ttscalerange = ppl("targettrafo", trafo_pipeop = PipeOpTargetTrafoScaleRange$new(),
graph = PipeOpLearner$new(LearnerRegrRpart$new()))
ttscalerange$train(task)
ttscalerange$predict(task)
ttscalerange$state$regr.rpart
}
#> $model
#> n= 506
#>
#> node), split, n, deviance, yval
#> * denotes terminal node
#>
#> 1) root 506 21.0260400 0.3895301
#> 2) town=Arlington,Ashland,Beverly,Boston Allston-Brighton,Boston Charlestown,Boston Dorchester,Boston Downtown,Boston East Boston,Boston Forest Hills,Boston Hyde Park,Boston Mattapan,Boston North End,Boston Roxbury,Boston Savin Hill,Boston South Boston,Boston West Roxbury,Braintree,Burlington,Cambridge,Chelsea,Danvers,Dedham,Everett,Framingham,Hamilton,Hanover,Holbrook,Hull,Lynn,Malden,Marshfield,Medford,Melrose,Middleton,Millis,Nahant,Natick,Norfolk,North Reading,Norwell,Norwood,Peabody,Pembroke,Quincy,Randolph,Reading,Revere,Rockland,Salem,Sargus,Scituate,Sharon,Somerville,Stoneham,Wakefield,Walpole,Waltham,Watertown,Weymouth,Wilmington,Winthrop,Woburn 400 7.5706490 0.3174944
#> 4) lstat>=14.4 176 1.6065670 0.2189646
#> 8) town=Boston Charlestown,Boston East Boston,Boston Forest Hills,Boston North End,Boston Roxbury,Boston Savin Hill,Boston South Boston,Chelsea,Lynn 93 0.4849460 0.1569654 *
#> 9) town=Arlington,Beverly,Boston Allston-Brighton,Boston Dorchester,Boston Downtown,Boston Hyde Park,Boston Mattapan,Cambridge,Everett,Framingham,Malden,Medford,Middleton,Norwood,Peabody,Quincy,Revere,Salem,Somerville,Waltham,Watertown 83 0.3635828 0.2884337 *
#> 5) lstat< 14.4 224 2.9129590 0.3949107
#> 10) lstat>=4.63 215 0.9995600 0.3787494
#> 20) lstat>=7.765 150 0.5226398 0.3547556 *
#> 21) lstat< 7.765 65 0.1912833 0.4341197 *
#> 11) lstat< 4.63 9 0.5157443 0.7809877 *
#> 3) town=Bedford,Belmont,Boston Back Bay,Boston Beacon Hill,Brookline,Canton,Cohasset,Concord,Dover,Duxbury,Hingham,Lexington,Lincoln,Lynnfield,Manchester,Marblehead,Medfield,Milton,Needham,Newton,Sherborn,Sudbury,Swampscott,Topsfield,Wayland,Wellesley,Wenham,Weston,Westwood,Winchester 106 3.5470870 0.6613627
#> 6) rm< 7.437 82 1.5639410 0.5961518
#> 12) crim< 4.12641 75 0.6617538 0.5730963
#> 24) rm< 6.727 27 0.1925600 0.4869959 *
#> 25) rm>=6.727 48 0.1564460 0.6215278 *
#> 13) crim>=4.12641 7 0.4351788 0.8431746 *
#> 7) rm>=7.437 24 0.4430451 0.8841667 *
#>
#> $log
#> Empty data.table (0 rows and 3 cols): stage,class,msg
#>
#> $train_time
#> [1] 0.009
#>
#> $param_vals
#> $param_vals$xval
#> [1] 0
#>
#>
#> $task_hash
#> [1] "47ff66d9074b461c"
#>
#> $feature_names
#> [1] "age" "b" "chas" "crim" "dis" "indus" "lat"
#> [8] "lon" "lstat" "nox" "ptratio" "rad" "rm" "tax"
#> [15] "town" "tract" "zn"
#>
#> $validate
#> NULL
#>
#> $mlr3_version
#> [1] ‘0.20.0’
#>
#> $data_prototype
#> Empty data.table (0 rows and 18 cols): cmedv.scaled,age,b,chas,crim,dis...
#>
#> $task_prototype
#> Empty data.table (0 rows and 18 cols): cmedv.scaled,age,b,chas,crim,dis...
#>
#> $train_task
#> <TaskRegr:boston_housing> (506 x 18): Boston Housing Prices
#> * Target: cmedv.scaled
#> * Properties: -
#> * Features (17):
#> - dbl (12): age, b, crim, dis, indus, lat, lon, lstat, nox, ptratio,
#> rm, zn
#> - int (3): rad, tax, tract
#> - fct (2): chas, town
#>
#> attr(,"class")
#> [1] "learner_state" "list"