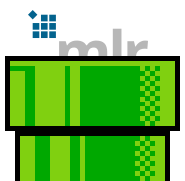
Change the Threshold of a Classification Prediction
Source:R/PipeOpThreshold.R
mlr_pipeops_threshold.Rd
Change the threshold of a Prediction
during the predict
step.
The incoming Learner
's $predict_type
needs to be "prob"
.
Internally calls PredictionClassif$set_threshold
.
Construction
id
::character(1)
Identifier of the resulting object, default"threshold"
.param_vals
:: namedlist
List of hyperparameter settings, overwriting the hyperparameter settings that would otherwise be set during construction. Defaults tonumeric(0)
.
Input and Output Channels
During training, the input and output are NULL
.
A PredictionClassif
is required as input and returned as output during prediction.
State
The $state
is left empty (list()
).
Parameters
thresholds
::numeric
A numeric vector of thresholds for the different class levels. May have length 1 for binary classification predictions, must otherwise have length of the number of target classes; seePredictionClassif
's$set_threshold()
method. Initialized to0.5
, i.e. thresholding for binary classification at level0.5
.
Fields
Fields inherited from PipeOp
, as well as:
predict_type
::character(1)
Type of prediction to return. Either"prob"
(default) or"response"
. Setting to"response"
should rarely be used; it may potentially save some memory but has no other benefits.
Methods
Only methods inherited from PipeOp
.
See also
https://mlr-org.com/pipeops.html
Other PipeOps:
PipeOp
,
PipeOpEncodePL
,
PipeOpEnsemble
,
PipeOpImpute
,
PipeOpTargetTrafo
,
PipeOpTaskPreproc
,
PipeOpTaskPreprocSimple
,
mlr_pipeops
,
mlr_pipeops_adas
,
mlr_pipeops_blsmote
,
mlr_pipeops_boxcox
,
mlr_pipeops_branch
,
mlr_pipeops_chunk
,
mlr_pipeops_classbalancing
,
mlr_pipeops_classifavg
,
mlr_pipeops_classweights
,
mlr_pipeops_colapply
,
mlr_pipeops_collapsefactors
,
mlr_pipeops_colroles
,
mlr_pipeops_copy
,
mlr_pipeops_datefeatures
,
mlr_pipeops_decode
,
mlr_pipeops_encode
,
mlr_pipeops_encodeimpact
,
mlr_pipeops_encodelmer
,
mlr_pipeops_encodeplquantiles
,
mlr_pipeops_encodepltree
,
mlr_pipeops_featureunion
,
mlr_pipeops_filter
,
mlr_pipeops_fixfactors
,
mlr_pipeops_histbin
,
mlr_pipeops_ica
,
mlr_pipeops_imputeconstant
,
mlr_pipeops_imputehist
,
mlr_pipeops_imputelearner
,
mlr_pipeops_imputemean
,
mlr_pipeops_imputemedian
,
mlr_pipeops_imputemode
,
mlr_pipeops_imputeoor
,
mlr_pipeops_imputesample
,
mlr_pipeops_kernelpca
,
mlr_pipeops_learner
,
mlr_pipeops_learner_pi_cvplus
,
mlr_pipeops_learner_quantiles
,
mlr_pipeops_missind
,
mlr_pipeops_modelmatrix
,
mlr_pipeops_multiplicityexply
,
mlr_pipeops_multiplicityimply
,
mlr_pipeops_mutate
,
mlr_pipeops_nearmiss
,
mlr_pipeops_nmf
,
mlr_pipeops_nop
,
mlr_pipeops_ovrsplit
,
mlr_pipeops_ovrunite
,
mlr_pipeops_pca
,
mlr_pipeops_proxy
,
mlr_pipeops_quantilebin
,
mlr_pipeops_randomprojection
,
mlr_pipeops_randomresponse
,
mlr_pipeops_regravg
,
mlr_pipeops_removeconstants
,
mlr_pipeops_renamecolumns
,
mlr_pipeops_replicate
,
mlr_pipeops_rowapply
,
mlr_pipeops_scale
,
mlr_pipeops_scalemaxabs
,
mlr_pipeops_scalerange
,
mlr_pipeops_select
,
mlr_pipeops_smote
,
mlr_pipeops_smotenc
,
mlr_pipeops_spatialsign
,
mlr_pipeops_subsample
,
mlr_pipeops_targetinvert
,
mlr_pipeops_targetmutate
,
mlr_pipeops_targettrafoscalerange
,
mlr_pipeops_textvectorizer
,
mlr_pipeops_tomek
,
mlr_pipeops_tunethreshold
,
mlr_pipeops_unbranch
,
mlr_pipeops_updatetarget
,
mlr_pipeops_vtreat
,
mlr_pipeops_yeojohnson
Examples
library("mlr3")
t = tsk("german_credit")
gr = po(lrn("classif.rpart", predict_type = "prob")) %>>%
po("threshold", param_vals = list(thresholds = 0.9))
gr$train(t)
#> $threshold.output
#> NULL
#>
gr$predict(t)
#> $threshold.output
#> <PredictionClassif> for 1000 observations:
#> row_ids truth response prob.good prob.bad
#> 1 good bad 0.8767123 0.1232877
#> 2 bad bad 0.1388889 0.8611111
#> 3 good bad 0.8687090 0.1312910
#> --- --- --- --- ---
#> 998 good bad 0.8687090 0.1312910
#> 999 bad bad 0.3795620 0.6204380
#> 1000 good bad 0.7391304 0.2608696
#>