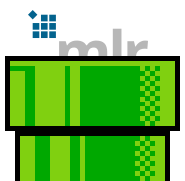
Linearly Transform Numeric Features to Match Given Boundaries
Source:R/PipeOpScaleRange.R
mlr_pipeops_scalerange.Rd
Linearly transforms numeric data columns so they are between lower
and upper
. The formula for this is \(x' = offset + x * scale\),
where \(scale\) is \((upper - lower) / (max(x) - min(x))\) and
\(offset\) is \(-min(x) * scale + lower\). The same transformation is applied during training and
prediction.
Format
R6Class
object inheriting from PipeOpTaskPreprocSimple
/PipeOpTaskPreproc
/PipeOp
.
Construction
id
::character(1)
Identifier of resulting object, default"scalerange"
.param_vals
:: namedlist
List of hyperparameter settings, overwriting the hyperparameter settings that would otherwise be set during construction. Defaultlist()
.
Input and Output Channels
Input and output channels are inherited from PipeOpTaskPreproc
.
The output is the input Task
with scaled numeric features.
State
The $state
is a named list
with the $state
elements inherited from PipeOpTaskPreproc
,
as well as the two transformation parameters \(scale\) and \(offset\) for each numeric
feature.
Parameters
The parameters are the parameters inherited from PipeOpTaskPreproc
, as well as:
lower
::numeric(1)
Target value of smallest item of input data. Initialized to 0.upper
::numeric(1)
Target value of greatest item of input data. Initialized to 1.
Methods
Only methods inherited from PipeOpTaskPreprocSimple
/PipeOpTaskPreproc
/PipeOp
.
See also
https://mlr-org.com/pipeops.html
Other PipeOps:
PipeOp
,
PipeOpEnsemble
,
PipeOpImpute
,
PipeOpTargetTrafo
,
PipeOpTaskPreproc
,
PipeOpTaskPreprocSimple
,
mlr_pipeops
,
mlr_pipeops_adas
,
mlr_pipeops_blsmote
,
mlr_pipeops_boxcox
,
mlr_pipeops_branch
,
mlr_pipeops_chunk
,
mlr_pipeops_classbalancing
,
mlr_pipeops_classifavg
,
mlr_pipeops_classweights
,
mlr_pipeops_colapply
,
mlr_pipeops_collapsefactors
,
mlr_pipeops_colroles
,
mlr_pipeops_copy
,
mlr_pipeops_datefeatures
,
mlr_pipeops_encode
,
mlr_pipeops_encodeimpact
,
mlr_pipeops_encodelmer
,
mlr_pipeops_featureunion
,
mlr_pipeops_filter
,
mlr_pipeops_fixfactors
,
mlr_pipeops_histbin
,
mlr_pipeops_ica
,
mlr_pipeops_imputeconstant
,
mlr_pipeops_imputehist
,
mlr_pipeops_imputelearner
,
mlr_pipeops_imputemean
,
mlr_pipeops_imputemedian
,
mlr_pipeops_imputemode
,
mlr_pipeops_imputeoor
,
mlr_pipeops_imputesample
,
mlr_pipeops_kernelpca
,
mlr_pipeops_learner
,
mlr_pipeops_missind
,
mlr_pipeops_modelmatrix
,
mlr_pipeops_multiplicityexply
,
mlr_pipeops_multiplicityimply
,
mlr_pipeops_mutate
,
mlr_pipeops_nmf
,
mlr_pipeops_nop
,
mlr_pipeops_ovrsplit
,
mlr_pipeops_ovrunite
,
mlr_pipeops_pca
,
mlr_pipeops_proxy
,
mlr_pipeops_quantilebin
,
mlr_pipeops_randomprojection
,
mlr_pipeops_randomresponse
,
mlr_pipeops_regravg
,
mlr_pipeops_removeconstants
,
mlr_pipeops_renamecolumns
,
mlr_pipeops_replicate
,
mlr_pipeops_rowapply
,
mlr_pipeops_scale
,
mlr_pipeops_scalemaxabs
,
mlr_pipeops_select
,
mlr_pipeops_smote
,
mlr_pipeops_smotenc
,
mlr_pipeops_spatialsign
,
mlr_pipeops_subsample
,
mlr_pipeops_targetinvert
,
mlr_pipeops_targetmutate
,
mlr_pipeops_targettrafoscalerange
,
mlr_pipeops_textvectorizer
,
mlr_pipeops_threshold
,
mlr_pipeops_tunethreshold
,
mlr_pipeops_unbranch
,
mlr_pipeops_updatetarget
,
mlr_pipeops_vtreat
,
mlr_pipeops_yeojohnson
Examples
library("mlr3")
task = tsk("iris")
pop = po("scalerange", param_vals = list(lower = -1, upper = 1))
task$data()
#> Species Petal.Length Petal.Width Sepal.Length Sepal.Width
#> <fctr> <num> <num> <num> <num>
#> 1: setosa 1.4 0.2 5.1 3.5
#> 2: setosa 1.4 0.2 4.9 3.0
#> 3: setosa 1.3 0.2 4.7 3.2
#> 4: setosa 1.5 0.2 4.6 3.1
#> 5: setosa 1.4 0.2 5.0 3.6
#> ---
#> 146: virginica 5.2 2.3 6.7 3.0
#> 147: virginica 5.0 1.9 6.3 2.5
#> 148: virginica 5.2 2.0 6.5 3.0
#> 149: virginica 5.4 2.3 6.2 3.4
#> 150: virginica 5.1 1.8 5.9 3.0
pop$train(list(task))[[1]]$data()
#> Species Petal.Length Petal.Width Sepal.Length Sepal.Width
#> <fctr> <num> <num> <num> <num>
#> 1: setosa -0.8644068 -0.9166667 -0.55555556 0.25000000
#> 2: setosa -0.8644068 -0.9166667 -0.66666667 -0.16666667
#> 3: setosa -0.8983051 -0.9166667 -0.77777778 0.00000000
#> 4: setosa -0.8305085 -0.9166667 -0.83333333 -0.08333333
#> 5: setosa -0.8644068 -0.9166667 -0.61111111 0.33333333
#> ---
#> 146: virginica 0.4237288 0.8333333 0.33333333 -0.16666667
#> 147: virginica 0.3559322 0.5000000 0.11111111 -0.58333333
#> 148: virginica 0.4237288 0.5833333 0.22222222 -0.16666667
#> 149: virginica 0.4915254 0.8333333 0.05555556 0.16666667
#> 150: virginica 0.3898305 0.4166667 -0.11111111 -0.16666667
pop$state
#> $Petal.Length
#> scale offset
#> 0.3389831 -1.3389831
#>
#> $Petal.Width
#> scale offset
#> 0.8333333 -1.0833333
#>
#> $Sepal.Length
#> scale offset
#> 0.5555556 -3.3888889
#>
#> $Sepal.Width
#> scale offset
#> 0.8333333 -2.6666667
#>
#> $dt_columns
#> [1] "Petal.Length" "Petal.Width" "Sepal.Length" "Sepal.Width"
#>
#> $affected_cols
#> [1] "Petal.Length" "Petal.Width" "Sepal.Length" "Sepal.Width"
#>
#> $intasklayout
#> Key: <id>
#> id type
#> <char> <char>
#> 1: Petal.Length numeric
#> 2: Petal.Width numeric
#> 3: Sepal.Length numeric
#> 4: Sepal.Width numeric
#>
#> $outtasklayout
#> Key: <id>
#> id type
#> <char> <char>
#> 1: Petal.Length numeric
#> 2: Petal.Width numeric
#> 3: Sepal.Length numeric
#> 4: Sepal.Width numeric
#>
#> $outtaskshell
#> Empty data.table (0 rows and 5 cols): Species,Petal.Length,Petal.Width,Sepal.Length,Sepal.Width
#>